November 2, 2022
Fullscreen ModeCongratulations to Kelly Heilman on the 2022 ESA Ecological Forecasting Award!
The ESA Statistical Ecology section is proud to present the 2022 Ecological Forecasting Outstanding Publication Award to Kelly Heilman and collaborators for their 2022 Global Change Biology Paper:
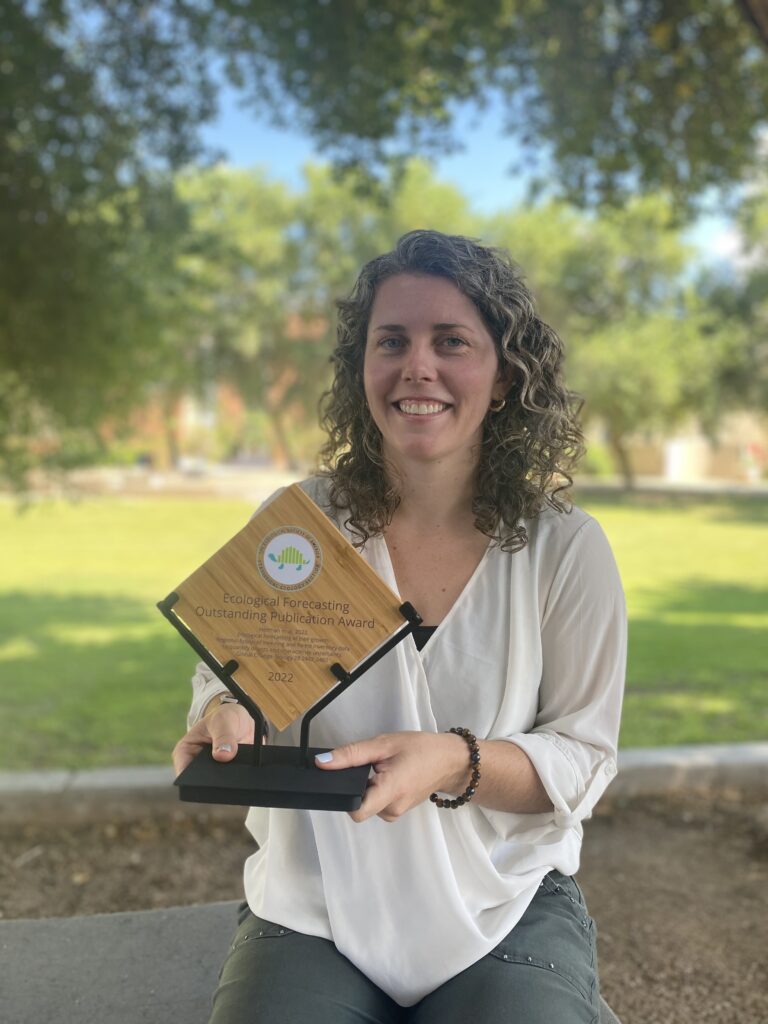
The award committee felt that the paper illustrates the strength of combining multiple data constraints across regional scales to improve predictions of forest growth for a climatically-vulnerable ecoregion, the American Southwest, parsing out the complex interactions among climate, stand, and individual-scale effects. Furthermore, the paper provides a detailed accounting of how different uncertainties impact growth projections across a range of time scales and climate projections, finding that tree growth and tree size were sensitive to very different uncertainties (year-to-year growth was dominated by driver uncertainty and process error, while tree size was more sensitive to initial conditions and plot random effects).
Individuals wishing to nominate papers published in the past 3 years for the 2023 award are encouraged to do so by the March 1, 2023 deadline. Additional information can be found at https://www.esa.org/stats/awards/ecological-forecasting-outstanding-publication-award/
Full List of Award Winners & Citations
2022 – Kelly Heilman (University of Arizona)
Heilman, K. A., Dietze, M. C., Arizpe, A. A., Aragon, J., Gray, A., Shaw, J. D., Finley, A. O., Klesse, S., DeRose, R. J., & Evans, M. E. K. (2022). Ecological forecasting of tree growth: Regional fusion of tree-ring and forest inventory data to quantify drivers and characterize uncertainty. Global Change Biology 28(7):2442-2460 doi.org/10.1111/gcb.16038
2021 – Sarah Saunders (National Audubon Society)
Saunders, S.P., F.J. Cuthbert, and E.F. Zipkin. “Evaluating Population Viability and Efficacy of Conservation Management Using Integrated Population Models.” Journal of Applied Ecology 55, no. 3 (2018): 1380–92. https://doi.org/10.1111/1365-2664.13080.
2020 – Paige Howell (USGS)
Howell, P.E., B.R. Hossack, E. Muths, B.H. Sigafus, A. Chenevert‐Steffler, and R.B. Chandler. “A Statistical Forecasting Approach to Metapopulation Viability Analysis.” Ecological Applications 30, no. 2 (2020): e02038. https://doi.org/10.1002/eap.2038.
2019 – Maria Paniw (CREAF, Ecological and Forestry Applications Research Centre)
Paniw, M., N. Maag, G. Cozzi, T. Clutton-Brock, and A. Ozgul. “Life History Responses of Meerkats to Seasonal Changes in Extreme Environments.” Science 363, no. 6427 (February 8, 2019): 631–35. https://doi.org/10.1126/science.aau5905.
2018 – Quinn Thomas (Virginia Tech)
Thomas, R.Q., E.B. Brooks, A.L. Jersild, E.J. Ward, R.H. Wynne, T.J. Albaugh, H. Dinon-Aldridge, et al. “Leveraging 35 Years of Pinus Taeda Research in the Southeastern US to Constrain Forest Carbon Cycle Predictions: Regional Data Assimilation Using Ecosystem Experiments.” Biogeosciences 14, no. 14 (2017): 3525–47. https://doi.org/10.5194/bg-14-3525-2017.
EFI Newsletter Issue 26
NEON Case Study Part 2 – The EFI-NEON Forecasting Challenge in the Classroom
September 8, 2022
See highlights about the NEON Forecasting Challenge in a two part series from the National Ecological Observatory Network blog.
This second post provides examples of how the NEON Forecasting Challenge has been used in undergraduate and graduate classes.
https://www.neonscience.org/impact/observatory-blog/efi-neon-forecasting-challenge-classroom
You can find the first post about building a forecasting community and individual experiences participating in the Challenge here:
EFI Newsletter Issue 25
EFI Futures Outstanding Student Presentation Award 2022 Results
At the May 2022 EFI Virtual Conference we debuted the EFI Futures Outstanding Student Presentation Award. This award is given to promote, recognize, and reward an outstanding student presenter and provides valuable feedback to student presenters on their research and presentation skills. Students presenting both Posters and Oral Presentations were eligible for this award. Each poster or oral presentation were anonymously reviewed by at least two volunteer reviewers with no conflicts of interest with the presenters. In addition to being recognized for their outstanding work, award winners received $50 to spend at the EFI shop. We thank all the students who presented and the volunteers who reviewed the presentations!
Congratulations to this year’s Outstanding Presentation Award recipients!
- Yiluan Song (University of California, Santa Cruz) won for her oral presentation, “Ecological forecasting of leafing and flowering phenology during climate change to inform public health” and
- Whitney Woelmer (Virginia Tech) won for her poster “Undergraduate student confidence and understanding of ecological forecasting concepts significantly increases after completing a Macrosystems EDDIE module.”
See Yiluan and Whitney’s presentation, poster and abstracts below.
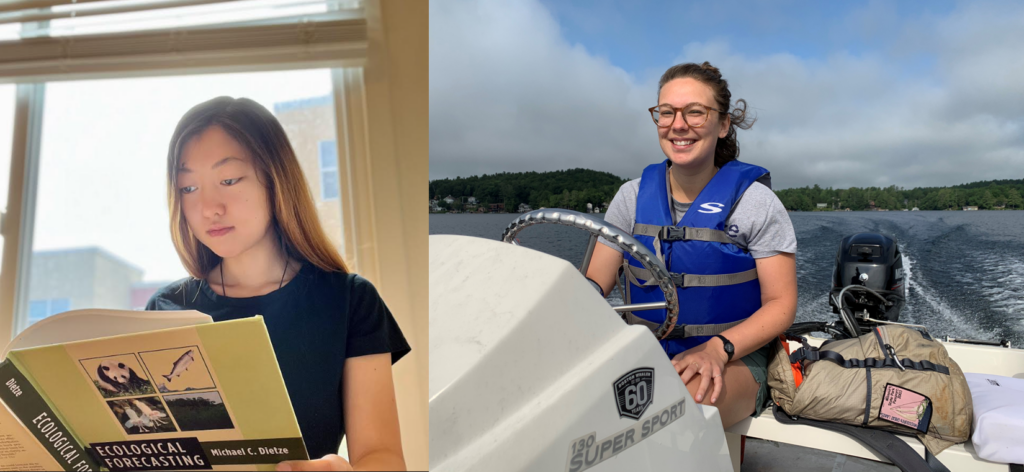
In her presentation, Yiluan introduced her pollen phenology forecast used for public health that leverages the rapidly accumulating data on leafing phenology with a nonlinear, data-driven model. Yiluan and her co-authors established a workflow for data assembly, prediction, and delivery and use the PhenoForecast platform to deliver forecasts of plant phenology and to communicate pollen allergy risks. The platform is not available online yet, but Yiluan and her collaborators are working toward that end.
In her poster, Whitney shared an overview of Module 8 of the Macrosystems EDDIE suite of educational modules that introduce students to concepts of macrosystems ecology, ecological forecasting, and quantitative literacy skills. This module in particular focuses on introducing decision-support and uncertainty communication skills, in addition to general ecological forecasting concepts and applications. Whitney’s poster also demonstrates how the effectiveness of the modules was assessed and how they increase students’ engagement and understanding of complex concepts.
Fullscreen ModeABSTRACTS
Ecological Forecasting of Leafing and Flowering Phenology During Climate Change to Inform Public Health
Yiluan Song1, Stephan B. Munch2,3,4, Kai Zhu1
1University of California, Santa Cruz, 2Southwest Fisheries Science Center, 3National Marine Fisheries Service, 4National Oceanic and Atmospheric Administration
Changes in plant phenology caused by climate change have important implications not only in ecology but also in public health. Global warming has led to an extended pollen season and increased pollen abundance. Exposure to pollen imposes significant costs on public health and is likely to exacerbate under future environmental change. The elevating risks from phenological shift calls for major improvements in the prediction of phenology and the understanding of driving mechanisms. The success in the prediction of phenology has been limited by the performance of phenology models, in particular, a mismatch between the common linear modeling approach and the nonlinear mechanisms. Traditional models with linear structures often fail to accurately predict phenology, as mechanisms of phenology can be highly nonlinear. Models that address nonlinear mechanisms with data-driven machine learning approaches are needed to overcome the limitations in phenology models. To model the nonlinear mechanisms of leafing and flowering phenology, we adopt a state-of-the-art machine learning method, Gaussian Process empirical dynamic modeling (GP-EDM). By forecasting leafing phenology observed with satellite remote sensing (MODIS) and near-surface camera imagery (PhenoCam) in the near term (as part of the EFI RCN NEON Ecological Forecast Challenge), we validate that our approach reveals complex causal relationships from time series and outperforms parametric alternatives in prediction. Applying our ecological forecasting method, we forecast the leafing and flowering phenology of four tree taxa that produce allergenic pollen at high spatial and temporal resolution (0.05 deg and daily) over the continental US. These forecasts, published daily through a Shiny App, serve to provide early allergy health advisories and warnings, which can limit pollen exposure and reduce healthcare costs. Further, the interactive query of plant phenology on the web app can raise awareness of the impacts of climate change on our health and the ecosystem. Overall, this project develops cutting-edge machine learning models for forecasting plant phenology and is a step towards mitigating the adverse impacts of unprecedented climate changes on public health.
Undergraduate Student Confidence and Understanding of Ecological Forecasting Concepts Significantly Increases after Completing a Macrosystems EDDIE Module
Whitney Woelmer1
1Virginia Tech
Ecological forecasting is an emerging approach to estimate future states of ecological systems with uncertainty, allowing society to prepare for and manage ecosystem services. Despite the increasing need to understand, create, and communicate forecasts, forecasting training has previously focused on graduate students, representing a gap in training undergraduate students as the next generation of ecologists. In response, we developed a hands-on teaching module within the Macrosystems EDDIE (Environmental Data-Driven Inquiry & Exploration; MacrosystemsEDDIE.org) educational program to introduce ecological forecasting and forecast communication to undergraduate students through an RShiny application. Tested with >250 undergraduate students, our assessment results suggest that the module significantly increased students’ ability to correctly define ecological forecasting terms, interpret forecast visualizations with uncertainty, and identify different ways to communicate forecast uncertainty for stakeholders. These results suggest that integrating ecological forecasting into undergraduate ecology curricula via software-based learning enhances students’ abilities to engage and understand complex ecological concepts.
NEON Case Study Part 1 – Building a Forecasting Community: The EFI-RCN NEON Forecasting Challenge
June 15, 2022
See highlights about the NEON Forecasting Challenge in a two part series from the National Ecological Observatory Network blog.
Find the first post about building a forecasting community and individual experiences participating in the Challenge here:
You can find the second post about how the NEON Forecasting Challenge has been used in the undergraduate and graduate classes here:
https://www.neonscience.org/impact/observatory-blog/efi-neon-forecasting-challenge-classroom