The Ecological Forecasting Initiative Bibliography Zotero Group library has an extensive list of citations. You can use the Filter Tags section in the lower left-hand corner of this library to search for papers tagged related to the following topics.
“EFI DEI Paper” – papers listed in the Bibliography linked on our EFI Diversity and Inclusion webpage
“EFI Slack Paper” – papers posted in the Slack #papers channel
“EFI Visualization Paper” – a compilation of resources for best practices for visualizing forecasts listed on our Social Science webpage
“EFI Partners & Knowledge Transfer Paper” – papers compiled by the Partners & Knowledge Transfer Working Group on co-production, team science, translational science, science communication, etc.
“EFI Publication” – publications that are specifically related to EFI activities and acknowledge an EFI funding source or are a product that came from an EFI working group
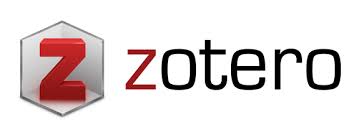
The following publications are categorized as:
EFI Publications, Concept Papers, Methods Papers, Decision Support, and Case Studies
The Concept, Methods, Decision Support, and Case Studies are examples that often were developed prior to the founding of EFI as a community.
EFI publications
* You can also find the EFI Publications on the EFI Google Scholar Page
These are publications that acknowledge an EFI funding source or are a product that came from an EFI working group or chapter
(Research Coordination Network, NSF funding, Alfred P. Sloan Foundation funding, EFI Education Working Group)
Lewis, A. S., Howard, D. W., Koren, G., Kowalski, C., McLachlan, J., Peters, J., Smies, G., Tabares, O. (2024). Ehics in ecological forecasting: four case-based teaching modules. EFI-RCN: Ecological Forecasting Initiative Research Coordination Network, QUBES Educational Resources. doi:10.25334/5D99-Y019. (This publication provides the same material published in Teaching Issues and Experiments in Ecology. However, the QUBES version allows others to share modifications they make to the materials and provides a DOI for the materials.)
Woelmer W.M., L.M. Bradley, L.T. Haber, D.H. Klinges, A.S.L. Lewis, E.J. Mohr, C.L. Torrens, K.I. Wheeler, A.M. Willson. 2021. Ten simple rules for training yourself in an emerging field. PLOS Computational Biology 17(10): e1009440. https://doi.org/10.1371/journal.pcbi.1009440 (NOTE: This publication was led and written entirely by EFI Student Association members.)
Dietze, M., C. Boettiger, A. Shiklomanov, Q. Thomas, & J. Peters. 2021. Ecological Forecasting Initiative: Community Standards R Package: v0.5 (Version v0.5). Zenodo. http://doi.org/10.5281/zenodo.7494824 (EFI Forecasting Standards Working Group)
Peters, JA. and R.Q. Thomas. 2021. Going virtual: What we learned from the Ecological Forecasting Initiative Research Coordination Network Virtual Workshop. Bulletin of the Ecological Society of America. https://doi.org/10.1002/bes2.1828
Meyers, M.F., et al. 2021. Virtual Growing Pains: Initial Lessons Learned from Organizing Virtual Workshop, Summits, Conferences, and Networking Events during a Global Pandemic. Limnology and Oceanography Bulletin. https://doi.org/10.1002/lob.10431
Sjodin, A., L. Haber, S. LaDeau, A. Willson, M. Lofton, T. Moore, O. Tabares-Mendoza, D. Dalbotten, J. McLachlan, M. Dietze, J. Peters. 2021. Ecological Forecasting Student Resource: Overview of Forecasting, Potential Careers, Helpful Courses. EFI-RCN: Ecological Forecasting Initiative Research Coordination Network, QUBES Educational Resources. doi:10.25334/8VRF-WS31 (EFI Education Working Group)
Tabares-Mendoza, O. 2021. Are you learning R? Suggestions to help you as you learn. EFI-RCN: Ecological Forecasting Initiative Research Coordination Network, QUBES Educational Resources. doi:10.25334/C477-BA02 (EFI Education Working Group)
Tabares-Mendoza, O. 2021. Are you teaching biostatistics with R? Suggestions to help you as work with your students.. EFI-RCN: Ecological Forecasting Initiative Research Coordination Network, QUBES Educational Resources. doi:10.25334/737B-R258 (EFI Education Working Group)
Willson, A. and J. Peters. 2021. Ecological Forecasting Repository of Online Educational Resources. EFI-RCN: Ecological Forecasting Initiative Research Coordination Network, QUBES Educational Resources. doi:10.25334/XAW7-JW16 (EFI Education Working Group and EFI Student Association)
Woodley, L. K. Pratt, S. Kobilka, J. Peters. 2021. CSCCE Community Profile: Ecological Forecasting Initiative. Zenodo. https://doi.org/10.5281/zenodo.5032317
Pennisi, E. 2019. An ecologist with an eye toward forecasting the future. ScienceInsider Interview.
Dietze, M. and H. Lynch. 2019. Forecasting a bright future for ecology. Frontiers in Ecology and the Environment. https://doi.org/10.1002/fee.1994
Dietze, M. C., et al. 2018. Iterative near-term ecological forecasting: Needs, opportunities, and challenges. Proceedings of the National Academy of Sciences 115(7): 1424-1432. https://doi.org/10.1073/pnas.1
Dietze, M. C., et al. 2017. NEON workshop report: Iterative near-term ecological forecasting: Needs, opportunities, and challenges. pp 72. doi:10.6084/m9.figshare.4715317
Non-EFI Papers
Concepts papers
Clark, J. S., et al. 2001. Ecological Forecasts: An Emerging Imperative. Science, 293: 657–60. DOI: 10.1126/science.293.5530.657
Currie, D.J. 2018. Where Newton might have taken Ecology. Global Ecology and Biogeography, 28: 18-27. https://doi.org/10.1111/geb.12842
Dietze M. C. 2017. Ecological Forecasting. Princeton University Press. 288 pp. ISBN 9781400885459
Dietze, M. C. 2017. Prediction in ecology: a first-principles framework. Ecological Applications, 27: 2048-2060. https://doi.org/10.1002/eap.1589
Harris, D. J., S. D. Taylor, and E. P. White. 2018. Forecasting biodiversity in breeding birds using best practices. PeerJ 6:e4278; https://doi.org/10.7717/peerj.4278
Houlahan J.E., et al. 2017. The priority of prediction in ecological understanding. Oikos 126: 1–7. https://doi.org/10.1111/oik.03726
Luo, Y., T. F. Keenan, and M. Smith. 2014. Predictability of the Terrestrial Carbon Cycle. Global Change Biology, 21(5): 1737–51. https://doi.org/10.1111/gcb.12766
Luo, Y., et al. 2011. Ecological forecasting and data assimilation in a data-rich era. Ecological Applications, 21(5): 1429-1442. https://doi.org/10.1890/09-1275.1
MacCracken, M. 2001. Prediction versus Projection—Forecast versus Possibility. WeatherZine Guest Editorial, 26: 3–4.
Petchey, O. L., et al. 2015. The ecological forecast horizon, and examples of its uses and determinants. Ecology Letters, 18: 597-611. https://doi.org/10.1111/ele.12443
Shuman, F.G. 1989. History of numerical weather prediction at the National Meteorological Center. Weather Forecast, 4:286–296. https://doi.org/10.1175/1520-0434(1989)004%3C0286:HONWPA%3E2.0.CO;2
Weng, E., and Y. Luo. 2011. Relative Information Contributions of Model vs. Data to Short- and Long-Term Forecasts of Forest Carbon Dynamics. Ecological Applications, 21: 1490–1505. https://doi.org/10.1890/09-1394.1
Methods Papers
Clark, J. S. 2005. Why Environmental Scientists Are Becoming Bayesians. Ecology Letters, 8: 2–14. https://doi.org/10.1111/j.1461-0248.2004.00702.x
Conn, P. B., et al. 2018. A Guide to Bayesian Model Checking for Ecologists. Ecological Monographs. http://doi.org/doi:10.1002/ecm
Dormann, C. F., et al. 2018. Model averaging in ecology: a review of Bayesian, information‐theoretic and tactical approaches for predictive inference. Ecological Monographs, 88(4); 485-504. https://doi.org/10.1002/ecm.1309
Doucet, A., and A. M. Johansen. 2011. A tutorial on particle filtering and smoothing: fifteen years later. In D. Crisan & B. Rozovskii (Eds.), The Oxford Handbook of Nonlinear Filtering (Vol. 12, pp. 656–704). Oxford University Press. https://www.seas.harvard.edu/courses/cs281/papers/doucet-johansen.pdf
Evensen, G. 2009b. The Ensemble Kalman Filter for Combined State and Parameter Estimation. IEEE Control Syst. Mag., 29 (3): 83–104.
Hooten, M., and N. T. Hobbs. 2015. A guide to Bayesian model selection for ecologists. Ecological Monographs, 85:3–28. https://doi.org/10.1890/14-0661.1
Hyndman, RJ and G. Athanasopoulos. 2018. Forecasting: principles and practice. OTexts, 2nd edition, 382 pp. ISBN 978-0987507112
Medlyn, B. E., et al. 2015. Using ecosystem experiments to improve vegetation models. Nature Climate Change, 5(6): 528–534. http://doi.org/10.1038/nclimat
van Oijen, M. 2017. Bayesian methods for quantifying and reducing uncertainty and error in forest models. Current Forestry Reports, 3(4): 269–280. http://doi.org/10.1007/s40725-
Wikle, C., and L. Berliner. 2007. A Bayesian tutorial for data assimilation. Physica D: Nonlinear Phenomena, 230(1–2): 1-16. http://doi.org/10.1016/j.physd
Decision Support
Bradford, J. B., et al. 2018. Anticipitory natural resource science and management for a dynamic future. Frontiers in Ecology and the Environment. http://doi.org/10.1002/fee.180
Gregory, R., et al. 2012. Structured Decision Making: A Practical Guide to Environmental Management Choices. Wiley-Blackwell. 312 pp. ISBN: 978-1-444-33341-1
Hobday, A.J., et al. 2019. Ethical considerations and unanticipated consequences associated with ecological forecasting for marine resources. ICES Journal of Marine Science. https://doi.org/10.1093/icesjms/fsy210
Ketz, A. C., et al. 2016. Informing management with monitoring data: the value of Bayesian forecasting. Ecosphere, 7(11): 01587. http://doi.org/10.1002/ecs2.15
Milly, P.C.D., et al. 2008. Stationarity Is Dead: Whither Water Management? Science, 319: 573–74. DOI: 10.1126/science.1151915
Morgan, M. G. 2014. Use (and Abuse) of Expert Elicitation in Support of Decision Making for Public Policy. Proceedings of the National Academy of Science, U.S.A., 111: 7176–84. https://doi.org/10.1073/pnas.1319946111
Williams, P. J., and M. B. Hooten. 2016. Combining statistical inference and decisions in ecology. Ecological Applications, 26:1930–1942. https://doi.org/10.1890/15-1593.1
Case Studies
Howell, P. E., B. R. Hossack, E. Muths, B. H. Sigafus, A. Chenevert-Steffler, and R. B. Chandler. 2019. A statistical forecasting approach to metapopulation viability analysis. Ecological Applications 30:e02038. *Received 2020 Ecological Forecasting Oustanding Publication Award
Chen, Y., et al. 2011. Forecasting Fire Season Severity in South America Using Sea Surface Temperature Anomalies. Science, 334: 787–791. http://doi.org/10.1126/science
Hardegree, S.P., J.T. Abatzoglou, M.W. Brunson, M.J. Germino, K.C. Hegewisch, C.A. Moffte, D.S. Pilliod, B.A.Roundy, A.R. Boehm, G.R. Meredith. 2018. Weather-centric rangeland revegetation planning. Randeland Ecology & Management, 71(1): 1-11. https://doi.org/10.1016/j.rama.2017.07.003
Hobbs N.T., et al. 2015. State-space modeling to support management of brucellosis in the Yellowstone bison population. Ecological Monographs, 85:525–556. https://doi.org/10.1890/14-1413.1
Ibanez, I., et al. 2014. Integrated assessment of biological invasions. Ecological Applications, 24(1): 25–37. https://doi.org/10.1890/13-0776.1
Kuikka, S., J. Vanhatalo, and H. Pulkkinen. 2014. Experiences in Bayesian Inference in Baltic Salmon Management. Statistical Science, 29(1): 42–49. http://dx.doi.org/10.1214/13-STS431
LaDeau, S. L., et al. 2011. Data-Model Fusion to Better Understand Emerging Pathogens and Improve Infectious Disease Forecasting. Ecological Applications, 21: 1443–60. https://doi-org.proxy.library.nd.edu/10.1890/09-1409.1
Ong, J.B.S., et al. 2010. Real-time Epidemic Monitoring and Forecasting of H1N1-2009 Using Influenza-like Illness from General Practice and Family Doctor Clinics in Singapore. PLoS One, 5: e10036. https://doi.org/10.1371/journal.pone.0010036
Paniw, M. et al. 2019. Life history responses of meerkats to seasonal changes in extreme environments. Science, 363(6427): 631-635. DOI: 10.1126/science.aau5905 *Received 2019 Ecological Forecasting Oustanding Publication Award
Scales, K. L., et al. 2017. Fit to predict? Eco-informatics for predicting the catchability of a pelagic fish in near real time. Ecological Applications, 27(8): 2313–2329. http://doi.org/10.1002/eap.161
Thomas, R. Q., et al. 2017. Leveraging 35 years of Pinus taeda research in the southeastern US to constrain forest carbon cycle predictions: regional data assimilation using ecosystem experiments. Biogeosciences 14: 3525–3547.
*Received 2018 Ecological Forecasting Oustanding Publication Award
Tredennick, A. T., et al. 2016. Forecasting climate change impacts on plant populations over large spatial extents. Ecosphere, 7(10): e01525. http://doi.org/10.1002/ecs2.15