March 28, 2024
Fullscreen ModeEFI Newsletter Issue 39
From Communities to Topologies, Forecasting is a Social System
January 30, 2024
Post by: Nicholas R. Record, Tandy Center for Ocean Forecasting, Bigelow Laboratory for Ocean Sciences
“Does the removal of urchins and perrywinkles lead to brown water?”
This question is one of hundreds of hypotheses I’ve heard over the years working with community groups on forecasting projects. It’s one of the reasons that community-centered science is fun. The ideas I encounter in communities are far more wide ranging than what’s found among scientists, who are also interesting, but are often much more in lock-step with each other (McClenachan et al. 2022).
Questions and ideas that come from communities can broaden the scientific perspective, but they can also give an indication of what’s important to that community. To me, this resonates, at a time when we know science needs to be more equitable and inclusive. It’s especially pertinent to environmental forecasting, which can have direct impacts on communities.
So how can a community’s knowledge shape a forecasting system?
Thinking about this question took me down a strange rabbit hole recently. Or maybe it was more of a complex network of gopher holes. Or a termite mound. However esoteric, I popped out the other side with some new info.
The starting point was remembering that making a forecast is more than just solving a math problem. The techniques of forecasting might be learned in a quantitative context, but a forecasting system is a social system. As a social system, there can be complex social dynamics, like reflexivity and environmental justice (Record et al. 2021, Wilson et al. 2023). There’s lots of potential for unintended consequences and other ethical pitfalls if the social dimensions are brushed over (Boettiger 2022, Hobday et al. 2019). There are plenty of cases where well-intentioned forecasts have caused harm (see the aforementioned references).
But what does this forecasting / social system look like?
Most forecasting papers are mainly quantitative, but it’s common to include a figure that diagrams how the quantitative exercise–the forecasting algorithm–fits within a social context. For example, the “ecological forecasting cycle” traces steps from hypothesis generation to model building, uncertainty quantification, forecast generation and communication, assessment and updating, and back to hypothesis generation (Moore et al.2022). The cycle diagram codifies the implicit social system, which in turn shapes what we decide to forecast and how. This particular system has communication to and feedback from groups that are influenced by the forecasts (generally managers). The cycle is taught and learned by forecasters as a way to iteratively improve forecasting systems within their social contexts.
A cycle is not the only model for diagramming a forecasting system within its social network. Because I’m curious and a bit of a nerd, I recently reviewed a collection of papers with flowcharts diagramming their forecasting systems for ecological forecasting (Figure 1). You can see the “forecasting cycle” of Moore (2022) in the bottom center. There’s a range of configurations, including pure cycles, unidirectional flows, trees, meshes, nearly fully connected networks, and combinations of patterns. Personally, I like the one in the middle row, from Petchey, that looks like a flux capacitor.
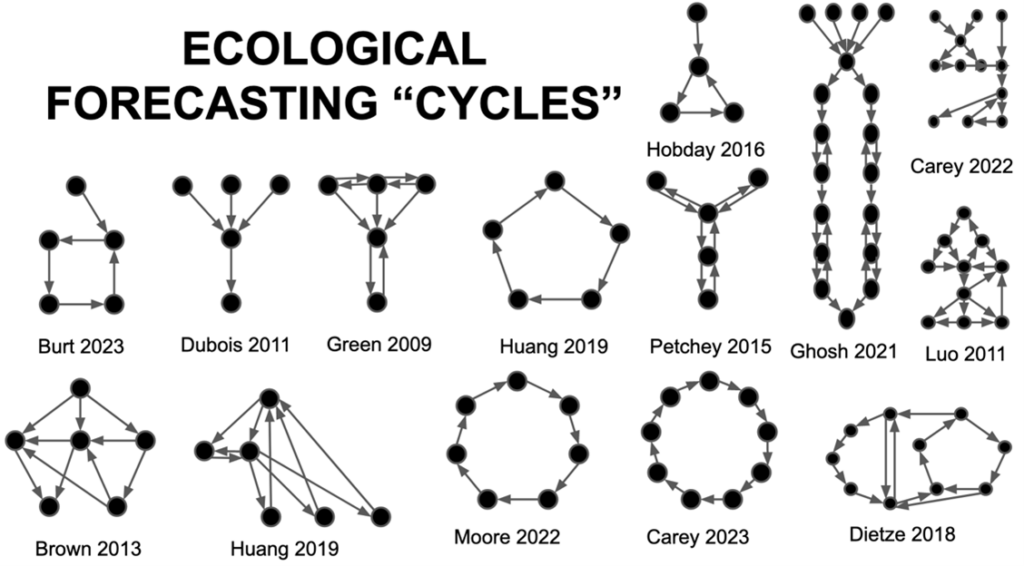
Figure 1 Some examples of topologies of ecological forecasting systems, diagrammed from the figures provided in a subset of the papers reviewed. Each figure was distilled as a network graph based on nodes and edges indicated in the diagram.
I think I used the word “esoteric” earlier, but these so-called topologies of networks can be pretty informative. The shape of a network influences the flow of information and the resultant emergent knowledge. For example, stronger connectivity leads to faster consensus, and under certain conditions, regular networks (same number of edges and nodes) can increase the probability of reaching a less biased consensus (Fernandes 2023).
Some patterns emerged from an analysis of this collection of networks. For example, diameter appears to increase with network size at a rate similar to that for cyclic networks–i.e. networks that are basically circular, like the “forecasting cycle” (Figure 2). To find the diameter of a network, you look at the shortest distances between all pairs of vertices, and take the largest of those (wikipedia explains it in more detail). In principle, larger networks don’t necessarily have to have larger diameters. Fully-connected networks have small diameters no matter how large, and other shapes (e.g. tree-like networks) fall somewhere in between. But for this group of papers, larger networks had larger diameters, which means information has to pass through many steps to get through the social network. Multiple steps can lead to situations where the information is not well connected with other parts of the system. One potential explanation for this is that as we build larger forecasting systems that include more social components, we have a tendency to overlook the importance of the connectivity of these components.
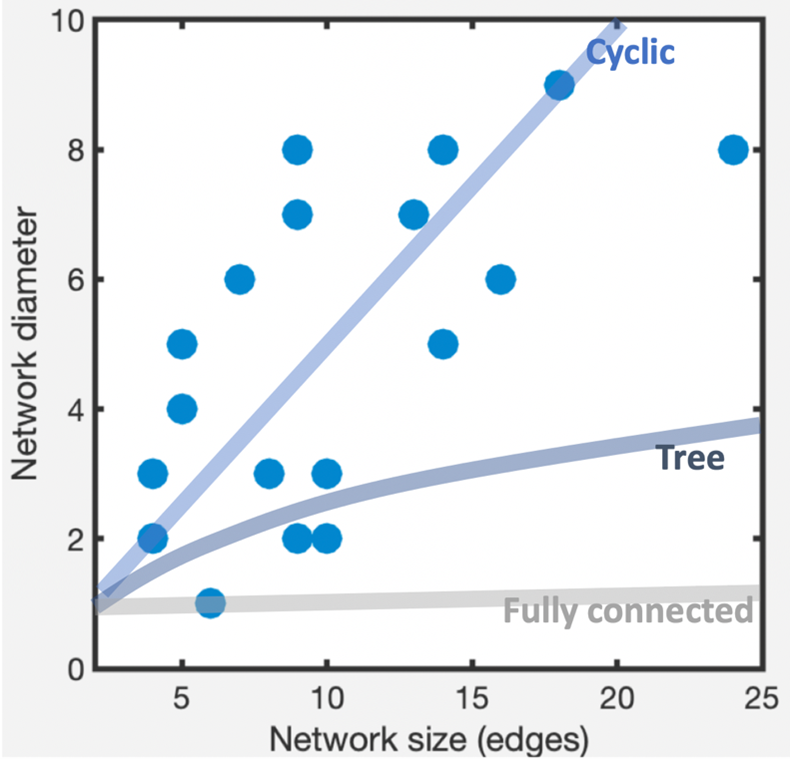
Figure 2 The relationship between network size (edges) and network diameter (greatest shortest node-node distance) across eighteen forecasting topologies (r = 0.64, p < 0.005). In comparison are lines showing what this relationship would look like for cyclic (bi-directional) networks, tree-like networks, and fully connected networks.
To put this knowledge to use, we redrew the schematics that we use for our own forecasting projects at the Tandy Center for Ocean Forecasting (Figure 3). The idea was to have a system that is fully connected, without long chains of arrows that information has to follow to get from one place to another. This exercise emerged through the process of writing this EFI blog post on barriers to inclusivity. The system has properties that aim to reduce confirmation bias and to speed consensus (Fernandes 2023)–i.e. full connectivity and regularity (equal number of nodes and vertices). In practice, the idea is to include forecast users and those influenced by forecast-based decisions–i.e. communities–as participants throughout the process. More details on this approach are in a technical document (Record 2022).
We don’t know for sure that our approach will lead to more equitable outcomes in forecasting. That’s just the working theory. There’s an important trend in ocean science (and many geosciences) developing the role of co-producing knowledge through collaborations across social systems (Liboiron et al. 2021, Schreiber et al. 2022). The schematic devised here seeks to address issues of accessibility to forecasting science and practice, though there are tradeoffs to this approach (Record et al. 2022).
Ideas like, “Does the removal of urchins and perrywinkles lead to brown water?” should be able to propagate quickly through a well-connected network and be incorporated, or not, in a forecasting system. By centering communities of people who use or are influenced by forecasts, it can help to kickstart forecasts in places that might be under-resourced or otherwise outside of the mainstream of ocean forecasting applications. It might help avoid unintended consequences of forecasts. And it should get a community’s wide range of hypotheses and ideas into the mix faster.
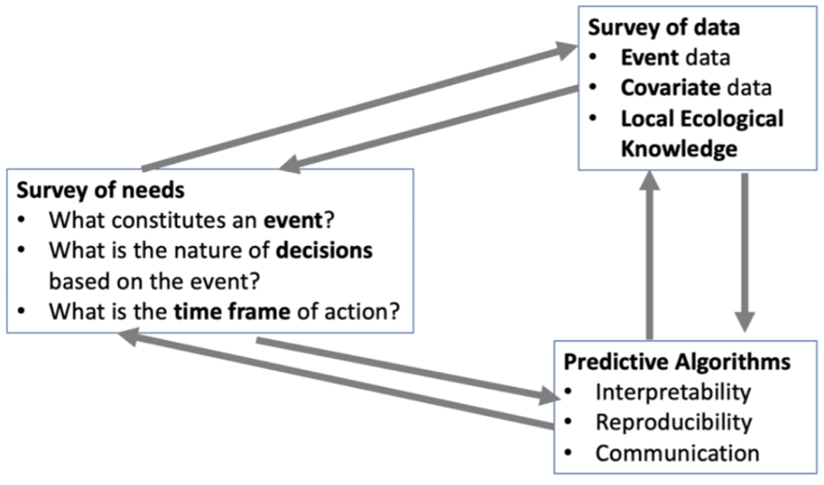
Figure 3 Schematic diagram of the framework used by the Tandy Center for building forecasting systems. Arrows indicate two-way movement of information between any of the components of the framework, potentially multiple times.
Do you have your own sketch of what a forecasting system network should look like? Or are you encouraged to try to make one? I suspect there are lots of different configurations that could work in different circumstances. If folks are willing to share, I’d be happy to collect them and post in a followup blog post. You can send it to forecast@bigelow.org, with a subject heading “topology”, or share on the EFI #inclusion Slack Channel (if you are not on the EFI Slack group and would like to join, reach out to info@ecoforecast.org to be added).
Note: Some of this content appeared in a blog post from 2023 (https://seascapescience.github.io/posts/2023/10/topologies/)
Acknowledgements: The EFI Diversity, Equity, and Inclusion Working Group provided helpful feedback on the post during the January 2024 call. Participants included: Alyssa Willson, Anna Sjodin, Antoinette Abeyta, Jason McLachlan, Jody Peters, John Zobitz, Rachel Torres, and Saeed Shafiei Sabet
References
Boettiger C. The forecast trap. Ecology Letters. 2022 Jul;25(7):1655-64. https://doi.org/10.1111/ele.14024
Fernandes MR. Confirmation bias in social networks. Mathematical Social Sciences. 2023 May 1;123:59-76. http://dx.doi.org/10.2139/ssrn.3504342
Hobday AJ, Hartog JR, Manderson JP, Mills KE, Oliver MJ, Pershing AJ, Siedlecki S. Ethical considerations and unanticipated consequences associated with ecological forecasting for marine resources. ICES Journal of Marine Science. 2019 Sep 1;76(5):1244-56. https://doi.org/10.1093/icesjms/fsy210
Liboiron M, Zahara A, Hawkins K, Crespo C, de Moura Neves B, Wareham-Hayes V, Edinger E, Muise C, Walzak MJ, Sarazen R, Chidley J. Abundance and types of plastic pollution in surface waters in the Eastern Arctic (Inuit Nunangat) and the case for reconciliation science. Science of the Total Environment. 2021 Aug 15;782:146809. https://doi.org/10.1016/j.scitotenv.2021.146809
McClenachan L, Record NR, Waller J. How do human actions affect fisheries? Differences in perceptions between fishers and scientists in the Maine lobster fishery. Facets. 2022 Feb 10;7(1):174-93. https://doi.org/10.1139/facets-2021-0030
Moore TN, Thomas RQ, Woelmer WM, Carey CC. Integrating ecological forecasting into undergraduate ecology curricula with an R shiny application-based teaching module. Forecasting. 2022 Jun 30;4(3):604-33. https://doi.org/10.3390/forecast4030033
Record NR, Pershing AJ. Facing the Forecaster’s Dilemma: Reflexivity in Ocean System Forecasting. Oceans 2021 Nov 12 (Vol. 2, No. 4, pp. 738-751). MDPI. https://doi.org/10.3390/oceans2040042
Record N. Early Warning Systems for Harmful Algae: A Stakeholder-Centered Framework. 2022. http://dx.doi.org/10.13140/RG.2.2.24501.14568
Record NR, Evanilla J, Kanwit K, Burnell C, Cartisano C, Lewis BJ, MacLeod J, Tupper B, Miller DW, Tracy AT, White C, Moretti M, Hamilton B, Barner C, Archer SD (2022) Benefits and Challenges of a Stakeholder-Driven Shellfish Toxicity Forecast in Coastal Maine. Frontiers in Marine Science. https://doi.org/10.3389/fmars.2022.923738
Schreiber MA, Chuenpagdee R, Jentoft S. Blue Justice and the co-production of hermeneutical resources for small-scale fisheries. Marine Policy. 2022 Mar 1;137:104959. https://doi.org/10.1016/j.marpol.2022.104959Willson AM, Gallo H, Peters JA, Abeyta A, Bueno Watts N, Carey CC, Moore TN, Smies G, Thomas RQ, Woelmer WM, McLachlan JS. Assessing opportunities and inequities in undergraduate ecological forecasting education. Ecology and Evolution. 2023 May;13(5):e10001. https://doi.org/10.1002/ece3.10001
EFI Newsletter Issue 38
EFI Newsletter Issue 37
EFI at AGU 2023
December 1, 2023
Below is the list of poster and oral presentations for EFI’s hosted session at the American Geophysical Union (AGU) 2022 Conference in Chicago, as well as other ecological forecasting-related talks that may be of interest to the community. All times are listed in US Pacific Time.
EFI has name badges! EFI community members can find Mike Dietze at the Conference, during the EFI-hosted sessions, or at the Social to get a badge.
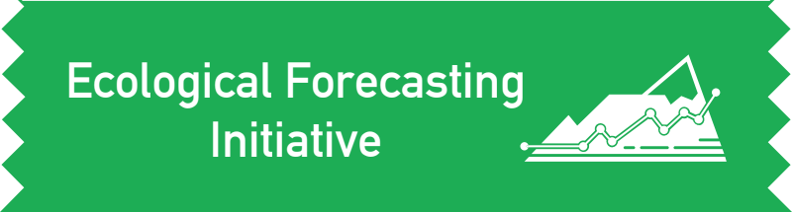
Tuesday EFI Social – Anyone who is available to meet up on December 12, Tuesday evening, we’ll have a group getting together from 7:00-9:00pm at 1015 Howard St, which is just an 11-minute walk from the Moscone Center.
Tuesday Poster and Oral Sessions – EFI’s oral and poster sessions on “Ecological Forecasting in the Earth System” will be held on Tuesday, December 12. The Poster Session is from 8:30am-12:50pm in Poster Hall A-C – South (Exhibition Level, South, MC). The Oral session is from 14:10-15:40pm in 3008 – West (Level 3, West, MC). We’re excited to have a great set of speakers that really span the full gradient from terrestrial to freshwater to marine. Come check out the following talks!
Tuesday Poster Session (9:00-12:30, Poster Hall A-C- South)
- Tierney Cantwell (Gettysburg College) – Sudden Oak Death and its Forecasted Threat to California Oaks using Maximum Entropy Modeling
- Joshua Ploshay (California State University Monterey Bay) – Spatiotemporal Patterns of Carbon (C) Cycle Variability Across North America: A Machine Learning Downscaling of a Process-based C Reanalysis Product
- Rob Kooper (University of Illinois at Urbana Champaign) – Unlocking the Power of ED2 Model on HPC Clusters: A Singularity Container Approach
- Qianyu Li (Brookhaven National Laboratory) – Assimilation of Satellite Observations Improves Seasonal Model Forecast of Leaf Area Index Over CONUS NEON Sites
- Matthew Petrie (University of Nevada Las Vegas) – Elucidating aridification pathways across the ecosystems of a semiarid elevational gradient
- Priyanka Singh (University of Petroleum and Energy Studies) – Machine Learning to Assess Large-scale Mining Impacts on Biodiversity Health
- Preethi Balaji (NASA DEVELOP) – Forecasting the Ecological Impacts of Targeted Cattle Grazing as a Management Tool to Improve Wetland Habitat in Southeastern Idaho
- Stephen E MacAvoy (American University) – Metabolic Plasticity, Measured by Stable Isotope Turnover, as a Proxy for Resilience to Warming Temperatures in the Seep Amphipod Gammarus minus and Isopod Caecidotea kenki
- Jiahao Zhang (Tsinghua University) – The origin of Risk of Golden Mussel (Limnoperna fortunei) Invasion
- Mark B Hausner (Desert Research Institute Reno) – Historical and Projected Drivers of Population Dynamics of the Endangered Devils Hole Pupfish (Cyprinodon diaboils)
Tuesday Oral Session (14:10-15:40, 3008 – West (Level 3, West, MC)
- 14:10 – Gerbrand Koren (Utrecht University) – Forecasting terrestrial carbon exchange over North America from sub-daily to multi-annual time scales
- 14:20 – Malcolm Itter (University of Massachusetts Amherst) – Making More With Continuous Forest Inventory Data: Toward a Scalable, Dynamical Model of Forest Change
- 14:30 – Alyssa Willson (University of Notre Dame) – Biotic interactions moderate the climate-vegetation relationship over the last 2,000 years of the pre-Industrial Holocene in the Upper Midwest, U.S.
- 14:40 – Morteza Karimzadeh (University of Colorado Boulder) – Forecasting Post-Fire Vegetation Recovery Using Deep Learning
- 14:50 – Dongchen Zhang (Boston University) – Terrestrial Carbon Pool Reanalysis Using State Data Assimilation Over CONUS NEON Sites
- 15:00 – Freya Olsson (Virginia Tech) – Power of multiple models in lake forecasting
- 15:10 – Dante Horemans (Virginia Institute of Marine Science, William & Mary) – Real-time Forecasts of Harmful Algal Blooms using Empirical Habitat Suitability Models
- 15:20 – Joshua Grail (ESRI) – Predicting Snow Crab Habitat Across Climate Scenarios Using Maximum Entropy Modelling
- 15:30 – Discussion
Other Forecasting Presentations
If you are presenting an ecological forecasting-related talk or poster that you don’t see on the list, reach out so we can get it added!
- Mon: 9:52-10:00, Anne Heggli, 2010 – West (Level 2, West, MC) – The Use of Hourly SNOTEL Data to Produce Snowpack Runoff Decision Support for Midwinter Rain-on-Snow
- Mon: 10:22-10:32, Isabella Kazen, 2007 – West (Level 2, West, MC) – Characterizing Parameter Uncertainty in Ecological Forecasts across Different Plant Functional Types
- Mon: 10:30-10:40, Michael Dietze, 2006 – West (Level 2, West, MC) – Landscape-scale iterative monitoring and forecasting of terrestrial carbon pools and fluxes: a Harvard Forest testbed
- Mon: 12:40-12:50, Ana M Tarano, 3009 – West (Level 3, West, MC) – Evaluating Global Benthic Habitat Maps Derived from Machine Learning: NASA’s Neural Multimodal Observation and Training Network
- Wed: 8:30-12:50, Yi Hong, Poster Hall A-C – South (Exhibition Level, South, MC) – Challenges and Opportunities for the Next Generation Water Resources Modeling Over the Laurentian Great Lakes Region
- Wed: 14:10-18:30, Xin Huang, Poster Hall A-C – South (Exhibition Level, South, MC) – Iterative forcing correction improves forecasts of carbon pool dynamics more than fluxes
- Wed: 14:10-18:30, Russell Limber, Poster Hall A-C – South (Exhibition Level, South, MC) – Forecasting River Ice Breakup in Alaska USA Using a Long Short Term Memory Model
- Thurs: 14:10-18:30, Enoch Bessah, Poster Hall A-C – South – Verification of Indigenous Weather and Seasonal Climate Forecast skills for Climate Change Adaptation in the Pra River Basin of Ghana
- Thurs: 15:50-16:15, Patrick J Clemins, 160 – South (Upper Mezzanine, South, MC) – Northeast Evaluation Testbeds for Hydrologic Impacts Forecasting
- Fri: 14:10-18:30, Pierre C Guillevic, Poster Hall A-C – South – Evaluation of Planet’s Biomass Proxy – a Combination of Optical and SAR Earth Observations – to Monitor Crop Conditions and Forecast Yield
EFI Book Club Suggestions & Actions Items
November 27, 2023
The following document was created by the DEI Working Group in October 2023 in response to the final discussion of the group that participated in the book club which read “97 Things About Ethics Everyone in Data Science Should Know”.
Goals of this document:
- Provide a summary of action items suggested during book clubs. The summary is a living document kept on the EFI Book Club webpage and action items will be added from future book clubs. This document is to be used as a reference to support and inspire activities for EFI that can be developed/led as individuals have time, availability, and interest in doing so
- Provide an opportunity to collate and celebrate activities that have taken place and those activities that will take place in the future that have been inspired by or align with suggestions from the book clubs.
Books Read
- 97 Things About Ethics Everyone in Data Science Should Know
- Weapons of Math Destruction
- Braiding Sweetgrass
- Caste: The Origins of Our Discontents
- The Years That Matter Most: How College Makes or Breaks Us
Note: There was a group within EFI that read Spatio-Temporal Statistics with R. That book group was not led by the DEI working group and was not included in this assessment.
Have we done any of the stuff we planned to do?
- From 97 Things About Ethics
- Summarize ideas we’ve had in previous book clubs and do self-assessment
- This document!
- Summarize ideas we’ve had in previous book clubs and do self-assessment
- From Braiding Sweetgrass
- Gratitude statement regarding data as a gift from the community, posted in:
- Newsletter
- Book club page
- The book club included student participants from the Sustainable Land and Water Resources (SLAWR) REU which provided opportunities for discussions across career stages and sectors
- Gratitude statement regarding data as a gift from the community, posted in:
- From Weapons of Math Destruction
- JEDI (justice, equity, diversity, inclusion) database assessing who is in EFI is a good place to start to contribute to the self-assessment
- Dave Klinges (University of Florida) has worked with EFI Community Manager, Jody Peters (University of Notre Dame) to develop a workflow to assess the demographics of EFI members and how that changes through time
- JEDI (justice, equity, diversity, inclusion) database assessing who is in EFI is a good place to start to contribute to the self-assessment
- From The Years That Matter Most and from Caste
- DEI Workshop ideas – webinar series leading up to workshops. Whose voices do we want to hear ahead of time
- Culturally competent training
- In late 2020/early 2021 the EFI DEI working group hosted 4 panels for the “Conversations on Diversity and Data Seminar Series.” See the links below for the recordings for each panel and the resources shared.
- What do diverse students need to thrive in data- and computer-heavy settings? November 19, 2020
- What does culturally competent data science training look like? January 20, 2021
- How can 4-year data-driven institutions and programs support students transferring from community colleges? February 19, 2021
- What kind of online tools are useful and work well for remote learning and teaching? March 25, 2021
- From Caste:
- Mentorship networks and having a series of mentors
- EFISA co-working session
- Developing a mentoring network for minority students is one of the goals of the Alfred P. Sloan Foundation Equitable Pathways funding from the project “A Generation of Native American Earth and Environmental Data Scientists” that EFI is involved with
- Still working on developing this, but the idea is for BIPOC students in research to have mentors from their own institution as well as mentors from the institutions they are doing research with and that the mentors will continue to support the students beyond the original activity
- Mentorship networks and having a series of mentors
- From the Years That Matter Most
- Train the trainers
- This is something that continues to be included in EFI-led proposals. Freya Olsson is also thinking about updating her NEON Forecasting Challenge workshop material which is currently geared towards participants wanting to learn how to submit forecasts to the Challenge to be applicable for people want to teach others how to submit to the Forecasting Challenge
- On the October 2023 Education working group call, the group discussed the following two papers. The first is about the needs for instructor training for people teaching data science at the collegiate level. The second provides papers, discussion prompts, and R code materials that can be used or modified for teaching ecological forecasting at the upper undergrad/early grad student level
- Highlight the #jobs and #funding Slack channels in the newsletter and in the welcome letter for new EFI members.
- Train the trainers
Do any of the ideas overlap between books?
- The Years That Matter Most, Caste, and 97 Things: mentorship, supporting involvement, inclusive trainings
- Ongoing EFI efforts with potential overlap:
- EFISA efforts to share grant applications
- Ongoing EFI efforts with potential overlap:
- Braiding Sweetgrass, Weapons of Math Destruction, and 97 Things: addressing unintended consequences of forecasts and taking time for self reflection
- Ongoing EFI efforts with potential overlap:
- Examining EFI efforts from the lens of Design Justice Principles
- Social scientist-ecologist matchmaking
- Ongoing EFI efforts with potential overlap:
A full list of the notes and recommendations compiled from the book clubs can be found in the living document on the EFI Book Club webpage.
EFI Newsletter Issue 36
Congratulations to Jacob Zwart on the 2023 ESA Ecological Forecasting Award!
The ESA Statistical Ecology section presented the 2023 Ecological Forecasting Outstanding Publication Award to Jacob Zwart and collaborators for their 2022 Journal of the American Water Resources Association paper:
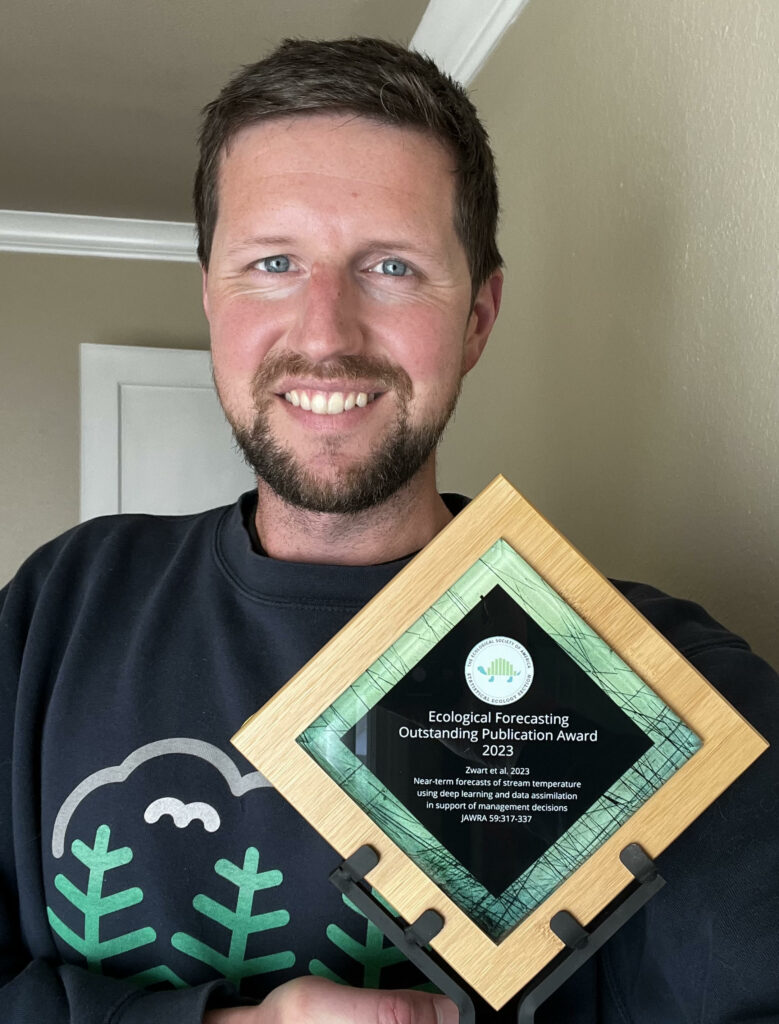
The award committee was impressed by the novel methodological contributions of the paper, in fusing machine learning with traditional data assimilation approaches. The committee also values the authors’ ability to put this forecast into operations, to tie a novel forecasting approach to actionable real-world decisions, and the overall readability and approachability of what is otherwise a very technical paper.
Nominate Papers for the 2024 Award
Individuals wishing to nominate papers published in the past 3 years for the 2024 award are encouraged to do so by the March 1, 2024 deadline. Additional information can be found at https://www.esa.org/stats/awards/ecological-forecasting-outstanding-publication-award/
Full List of Award Winners & Citations
2023 – Jacob Zwart (USGS)
Zwart, J.A., Oliver, S.K., Watkins, W.D., Sadler, J.M., Appling, A.P., Corson-Dosch, H.R., Jia, X., Kumar, V., and Read, J.S. 2023. “Near-Term Forecasts of Stream Temperature Using Deep Learning and Data Assimilation in Support of Management Decisions.” JAWRA Journal of the American Water Resources Association 59 (2): 317–37. https://doi.org/10.1111/1752-1688.13093.
2022 – Kelly Heilman (University of Arizona)
Heilman, K.A., Dietze, M.C., Arizpe, A.A., Aragon, J., Gray, A., Shaw, J.D., Finley, A.O., Klesse, S., DeRose, R.J., & Evans, M.E.K. (2022). Ecological forecasting of tree growth: Regional fusion of tree-ring and forest inventory data to quantify drivers and characterize uncertainty. Global Change Biology 28(7):2442-2460 doi.org/10.1111/gcb.16038
2021 – Sarah Saunders (National Audubon Society)
Saunders, S.P., F.J. Cuthbert, and E.F. Zipkin. “Evaluating Population Viability and Efficacy of Conservation Management Using Integrated Population Models.” Journal of Applied Ecology 55, no. 3 (2018): 1380–92. https://doi.org/10.1111/1365-2664.13080.
2020 – Paige Howell (USGS)
Howell, P.E., B.R. Hossack, E. Muths, B.H. Sigafus, A. Chenevert‐Steffler, and R.B. Chandler. “A Statistical Forecasting Approach to Metapopulation Viability Analysis.” Ecological Applications 30, no. 2 (2020): e02038. https://doi.org/10.1002/eap.2038.
2019 – Maria Paniw (CREAF, Ecological and Forestry Applications Research Centre)
Paniw, M., N. Maag, G. Cozzi, T. Clutton-Brock, and A. Ozgul. “Life History Responses of Meerkats to Seasonal Changes in Extreme Environments.” Science 363, no. 6427 (February 8, 2019): 631–35. https://doi.org/10.1126/science.aau5905.
2018 – Quinn Thomas (Virginia Tech)
Thomas, R.Q., E.B. Brooks, A.L. Jersild, E.J. Ward, R.H. Wynne, T.J. Albaugh, H. Dinon-Aldridge, et al. “Leveraging 35 Years of Pinus Taeda Research in the Southeastern US to Constrain Forest Carbon Cycle Predictions: Regional Data Assimilation Using Ecosystem Experiments.” Biogeosciences 14, no. 14 (2017): 3525–47. https://doi.org/10.5194/bg-14-3525-2017.